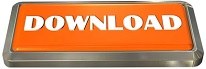
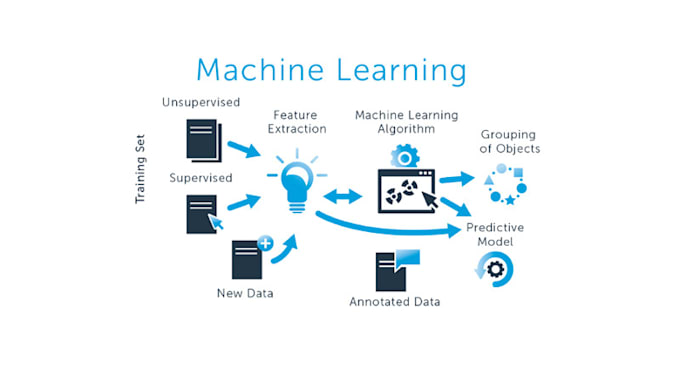
Naive Bayes is a fast and simple algorithm that is often used in applications such as spam filtering, sentiment analysis, and text classification. Gaussian Naive Bayes assumes that the features follow a Gaussian distribution, Multinomial Naive Bayes is used for discrete data such as text, and Bernoulli Naive Bayes is used for binary data. There are three main variants of Naive Bayes: Gaussian Naive Bayes, Multinomial Naive Bayes, and Bernoulli Naive Bayes. In the context of Naive Bayes, the algorithm is used for classification tasks, where the goal is to predict the class label of a new data point based on its features. Naive Bayes is a probabilistic machine learning algorithm based on Bayes' theorem, which describes the relationship between the probability of an event and the prior knowledge about that event. The method is easy to implement, computationally efficient, and has relatively low variance, making it a popular choice for many data scientists.
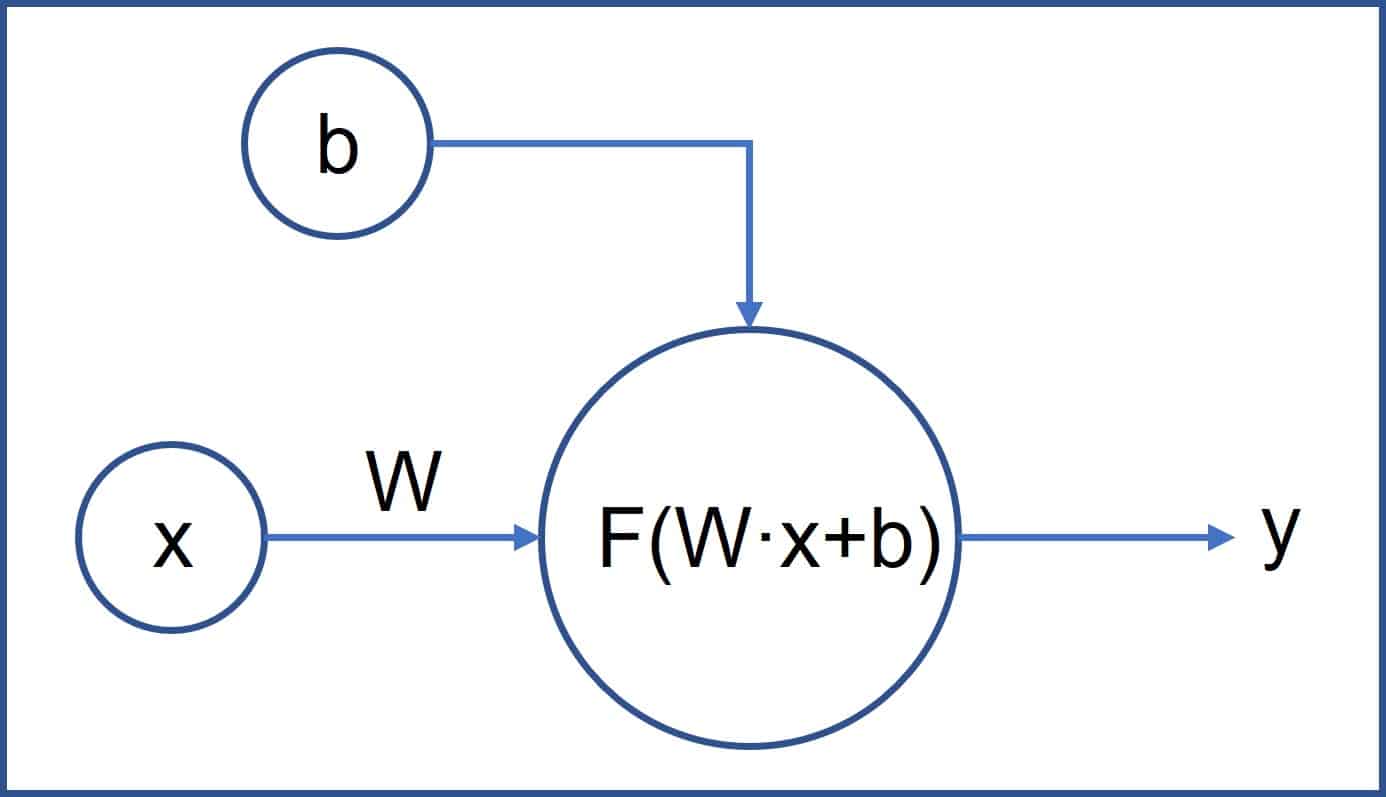
Logistic Regression can be used for a variety of applications, such as image classification, spam detection, and disease diagnosis, and is a fundamental technique in many machine learning algorithms. The logistic function models the odds of the positive class, rather than the probability directly. This probability value is then thresholded to make the binary prediction. In logistic regression, the relationship between the dependent and independent variables is modeled using the logistic function, which outputs a probability value between 0 and 1. Unlike linear regression, which is used for continuous outcomes, logistic regression is used for predicting binary outcomes, such as yes/no, true/false, or positive/negative.

Logistic Regression is a statistical method for analyzing a dataset in which there is a dependent variable and one or more independent variables. KNN is widely used in applications such as image classification, anomaly detection, and recommendation systems. However, it can be computationally expensive when making predictions on large datasets and may suffer from the curse of dimensionality, where the number of features becomes large relative to the number of samples. KNN is simple to implement, fast for small datasets, and has little to no training time, as the algorithm only stores the training data. For regression tasks, the prediction is made by averaging the values of the K nearest neighbors. For classification tasks, the prediction is made by voting among the K nearest neighbors, where the majority class is returned as the prediction. In KNN, the value of K is specified by the user and represents the number of nearest neighbors used to make a prediction for a new data point. The basic idea behind KNN is that the outcome of a data point is determined by its closest neighbors in the feature space. K-Nearest Neighbors (KNN) is a non-parametric machine learning algorithm used for both classification and regression tasks.
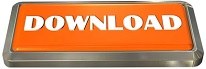